Understanding customer behaviour through user-generated data
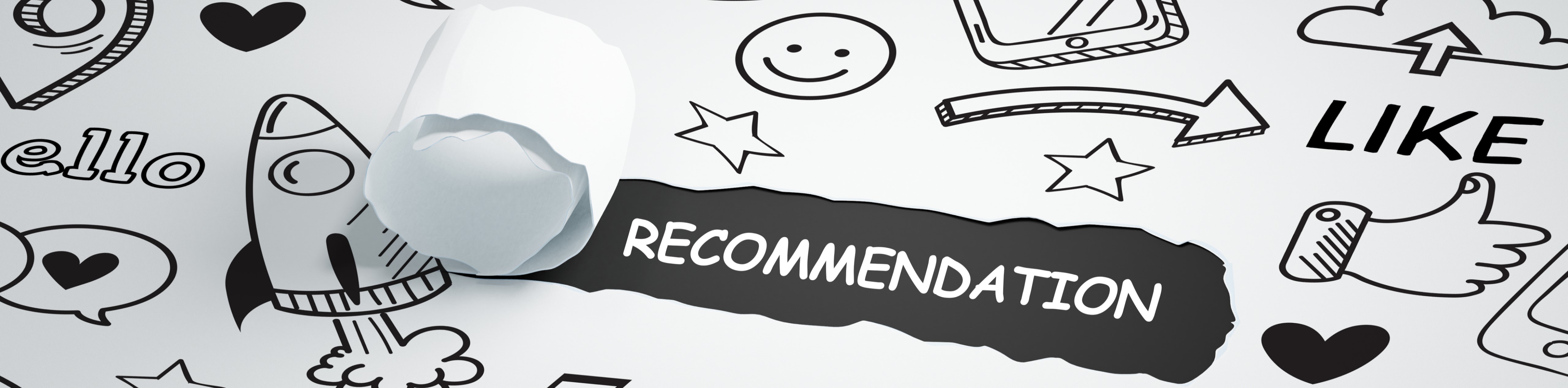
Ever felt overwhelmed when entering a mega-mall, populated by culinary treats, unbeatable bargains, and trending looks at every price point? Try expanding that smorgasbord of offerings immeasurably, and one could imagine why online shopping is at once a wondrous distraction and a black hole of potential purchases. Throw in a relentless calendar of sale events, product launches, event notifications and new friend requests, and going online becomes a decision-making delirium, whereby netizens are inundated by endless selections.
“The last decade has witnessed the rapid growth of e-applications such as Amazon, YouTube, Netflix, and Spotify,” notes Andrew Le Duy Dung, who was part of the pioneering batch of PhD in Computer Science graduates from SMU’s School of Information System in 2020 [now the School of Computing and Information Systems].
“However, as the number of products and online artefacts (for example, videos, images etc.) are growing exponentially, consumers also face the issue of choice explosion.”
With research interests that included Recommender Systems, Information Retrieval and Visual Analytics Research, Andrew is on a mission to improve the quality and the scalability of online recommendation systems like review sites.
“As many consumers are using these platforms for their daily shopping and entertainment, we can now understand their preferences better,” explains Andrew, who is now a senior data scientist with ride-hailing company Grab.
“By using machine learning and data mining techniques, personalised systems can filter out and suggest a small number of items that are curated towards the preference of each individual.”
Formerly a Research Scientist in SMU, Andrew’s PhD thesis focused on analysing diverse types of customer behaviours, towards generating high quality personalised recommendations for each individual.
“Such technology will help businesses to build and retain strong relationships with customers and drive loyalty. Customers can enjoy a unique experience whenever they go online.”
For example, over 80 per cent of shows streamed on Netflix are discovered by the content streaming giant’s recommendation system. By employing machine learning and complex algorithms informed by a user’s watching pattern, Netflix offers a curation of shows from its extensive catalogue in a bid to encourage more time spent on the platform.
“Personalisation systems have been studied extensively, but far from being solved,” adds Andrew. “My research focuses on improving the quality of the recommended items and guaranteeing that these systems are scalable, to the extent that they can serve millions of consumers at the same time.”
According to a 2021 report by market research firm Market Research Future, the global Recommendation Search Engine market is expected to reach approximately US$5.9 billion by 2023. This immense growth is fuelled by rapid digitalisation and the need to enhance customer experience, as well as surge in demand for the analysis of huge volumes of data. Which makes the work of Andrew and SMU research undertaking Preferred.AI, incredibly pertinent. The group, of which Andrew is part and comprises PhD students, research scientists, research engineers and visiting research students from SMU, pushes the envelope on learning user preferences from data to improve the effectiveness and efficiency of recommendations using data mining, machine learning, and artificial intelligence.
“I collaborated with my teammates at Preferred.AI to create open source tools and frameworks for several components of a recommender system,” elaborates Andrew. “These frameworks provide useful resources for researchers who are interested in the topics of recommender systems or preference analytics.”
The group’s work includes designing algorithms for mining user-generated data like ratings, text, images and social networks, to understand the behaviours and preferences of users, and apply the mined knowledge to develop user-centric intelligent applications.
“Companies can also use these frameworks to quickly experiment and assess the effectiveness of adopting personalisation into their businesses,” says Andrew.
With the digital economy sparked by the current stay-at-home culture, as the world continues to combat the pandemic, online customer experience is more of a priority than ever before. Hopefully, rather than tuning us into even greater options of entertainment, consumerism and information, the next generation of recommender systems will help narrow down our choices so we can regain one of our most precious assets – time.
Views expressed by Andrew Le Duy Dung in this article are his own and don't necessarily reflect those of his employer.
See also: Life as a Self-driven PhD Student in Multicultural Singapore