Breaking new ground with research into governance and policing
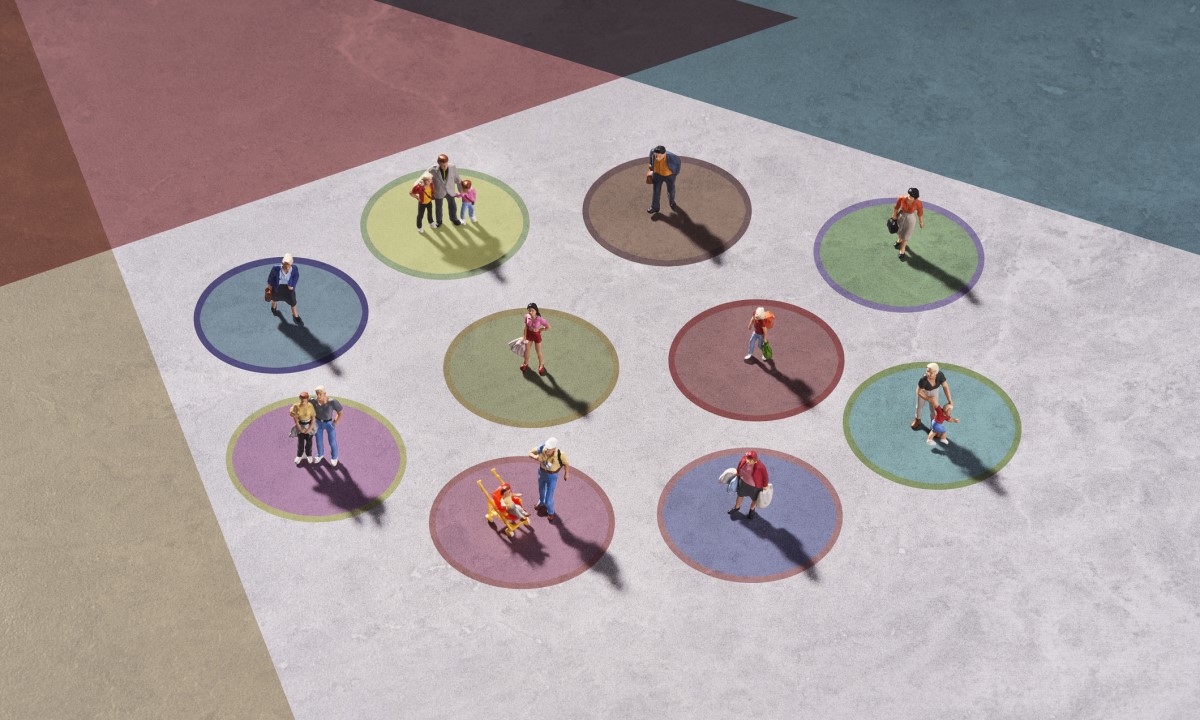
Research remains integral to our creation of sustainable yet liveable cities. Ideas from research can be informative to policymakers and help to change the way we understand societal issues and navigate problems.
SMU has continuously shown its commitment to applying its knowledge and global perspective in our Asian context in order to bridge global concepts and provide critical insights in to usable, local contexts. SMU’s students and faculty continue to display this spirit through the research that they do, which contributes to meaningful impact to society.
We looked at two recent studies that have received recognition for these efforts.
Boosting accountability in collaborative governance
Accountability is key to good governance – however, there are many intricacies in the accountability challenges in emerging contexts, especially when it comes to collaborative governance.
Take the Singaporean context, for example. There are multiple government bodies such as the National Environment Agency (NEA), which coordinates with the Ministry of the Environment and other ministries in order to pursue its green agenda. While different bodies specialise in an area of governance particular to themselves, it also creates complexities when it comes to maintaining accountability overall. And of course, information about enforcement standards, challenges and operational excellence must be shared across these agencies.
Even from such a structured example, it is clear how various societal actors from different sectors and industries have shared policy goals but are often working towards divergent directions in modern governance models.
Assistant Professor of Public Policy Seulki Lee from SMU’s School of Social Sciences (SOSS) sought to explore these complexities in “When Tensions Become Opportunities: Managing Accountability Demands in Collaborative Governance”, published in the Journal of Public Administration Research and Theory (JPART). JPART is one of the leading journals in the field of public administration.
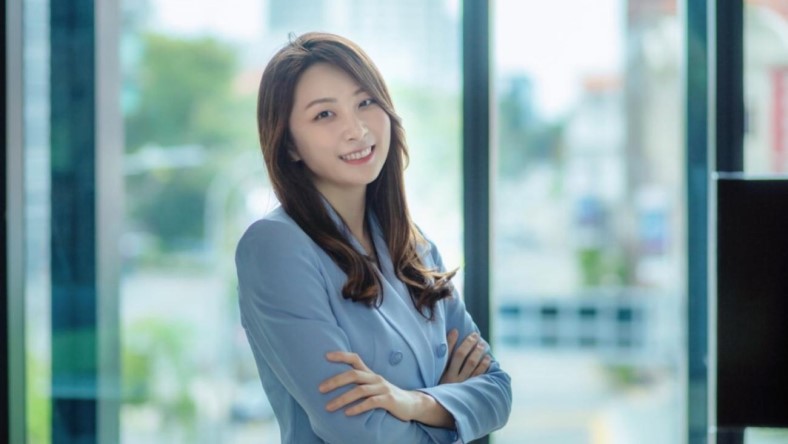
In order to provide insight into the complexities of maintaining accountability in cross-sector, multi-actor networks, Asst Prof Lee conducted a qualitative case study of a Korean inter-organisational network that was dedicated to enhancing tourist safety. Based on interviews with network actors and document analysis, the study reveals that participants in collaborative governance experience the conflicting expectations arising from different accountability relationships, which can be conceptualized as accountability tensions. These accountability tensions vary across the sources of the demand, and they are more likely to emerge when procedural and institutional arrangements display gaps, or when collaborators' levels of commitment diverge.
The study identifies two types of response strategies used to manage accountability tensions – conflict-driven strategies, which actually help to avoid conflict in the short-term, but do not prevent the tension from recurring; and mission-driven strategies, in which actors actively confront a tension to find a long-term solution associated with the network’s mission.
The findings from the study indicate that accountability tensions, when harnessed and actively managed with mission-driven strategies, can become opportunities to enhance a network’s accountability and improve processes, advancing its mission.
The study goes on to propose a novel framework for accountability tensions in collaborative governance, and specific mechanisms through which such tensions can be managed.
Asst Prof Lee says, “My research aims to address a critical gap in the public management literature on accountability in emerging governance contexts by unravelling the complexities entailed in maintaining accountability within cross-sector, multi-stakeholder networks.”
Machine-learning scheduling of police presence
The second piece of research was in developing an innovative solution to keeping a good balance between having police presence in the community without it being disruptive,and being prompt in an emergency response.
Mr Wong Songhan, a master of IT in Business (MITB) Artificial Intelligence student at SMU’s School of Computing and Information Systems (SCIS) tackled this complex problem as part of his research capstone project titled “Dynamic Police Patrol Scheduling with Multi-Agent Reinforcement Learning”.
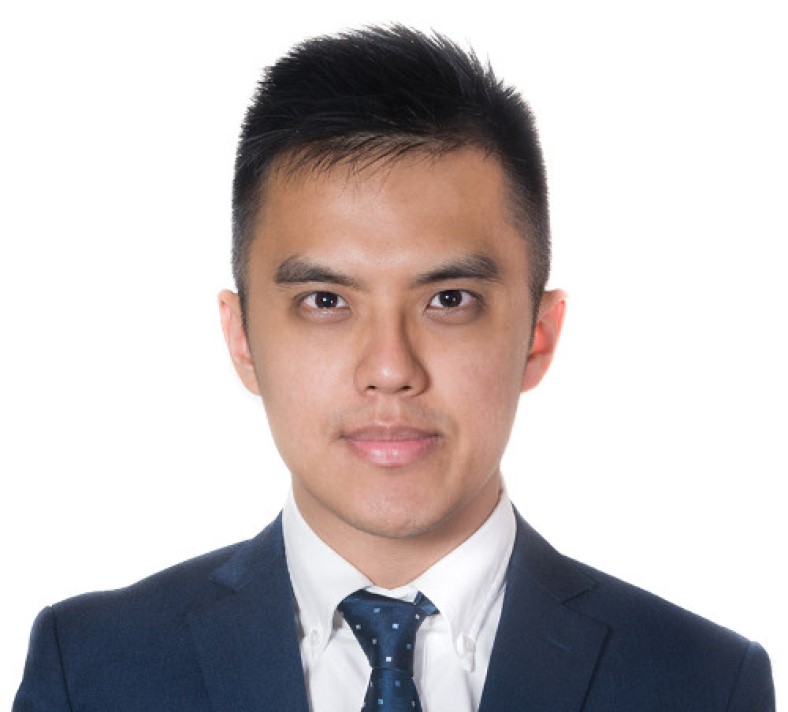
“Police patrol scheduling is complex because of the dynamic occurrences of incidents that require police’s attention and responding to an incident means that existing patrol plan will inevitably be disrupted and require almost instantaneous re-planning,” explains Mr Waldy Joe, a PhD student from SCIS who provided guidance for the research project.
“In this research, we wanted to train a machine to be able to [make] better decisions within operationally reasonable time.”
Supervised by Professor of Computer Science Lau Hoong Chin, Mr Wong used the Asynchronous Proximal Policy Optimisation-based (APPO) actor-critic method. APPO is a Reinforcement Learning (RL) algorithm where the machine is trained to learn by trial and error and by observing “rewards”. During the training period, the model is given a high reward for actions that are good, and low rewards for actions that are bad.
This approach allowed Mr Wong to train a machine that could then be used to dynamically reschedule existing patrol plans by learning a set of prescribed dispatch rules. The result not only reduces computational training time, but also improves the effectiveness of planned police patrols.
Mr Wong’s research paper was accepted for publication at the 17th Learning and Intelligent Optimisation Conference (LION17), an interdisciplinary conference in July 2023 that brought together experts in operations research, machine learning and related fields. The conference paper will also be published here.